Model Based Fault Diagnosis
Participating Associate Professor: Erik
Frisk.
Participating Assistant Professors: Mattias Krysander,
Mattias Nyberg, and Jan Åslund.
Projects are financed through Swedish Research Council, Programrådet för fordonsforskning, TurboPower, MOVIII, CENIIT, VISIMOD, and NFFP4.
Industrial partners are Sörman, Saab Aerosystems AB, Siemens Industrial Turbomachinery, Saab Automobile AB, Mecel AB, ABB Automation Systems, Scania and Volvo Technology.
Are you a student, interested in doing a master thesis in diagnosis? Take a look at our master thesis page (in Swedish) or contact us for further information.
What is Diagnosis?
From a general perspective, including both the medical and technical case, diagnosis can be explained as follows. For a process there are observed variables or behaviors for which there is knowledge of what is expected or normal. The task of diagnosis is to, from the observations and the knowledge, generate a diagnosis, i.e. to decide whether there is a fault or not and also to identify the fault. The following picture shows the information flow including a process and a diagnosis system.
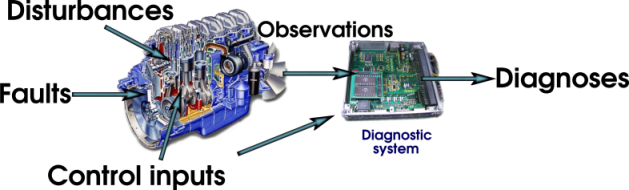
For a more elaborate introduction, see our popular introduction to diagnosis.
Diagnosis Research at Vehicular Systems
Diagnosis research at the group is currently performed within a few areas. Below is a brief description of these topics together with links to publications within each area.- Design and analysis of linear residual generators [Publications]
- Design and analysis of non-linear residual generators [Publications]
- Fault isolation [Publications]
- Structural methods [Publications]
- Applications [Publications]
A complete list of our diagnosis publications is found from the groups main publication page.
Design and analysis of linear residual generators
In any diagnosis system it is necessary to generate fault sensitive signals, residuals, with which the diagnosis system can draw conclusions about possible fault states of the supervised process. The aim of our research within residual generation is to develop methods to generate such residuals based on a mathematical description of the process. Fundamental difficulties are noise and uncertainties in the model descriptions and a main objective is to systematically handle these problems.
The problem is highly dependent on the type of mathematical
description of the process. A well studied class of models, for which
far reaching results are possible, are linear dynamic models. We have
studied how process models consisting of ordinary differential
equations (ODE), or more general differential-algebraic equations
(DAE) can be used to generate residuals and analyse detectability
etc. Both cases when the model is assumed perfect and uncertain has
been studied. Uncertain models mean models subjected to stochastic
noise or parametric uncertainties. In those cases, the design may end
up in an optimization problem where a trade-off is done between fault
sensitivity and sensitivity to the model uncertainties.
Publications
Design and analysis of non-linear residual generators
Nearly all systems exhibit non-linear behavior and to get optimal
performance of the diagnosis system, all system knowledge need to be
taken into account during design. Thus, it is desirable that also
non-linear process models can be considered when designing residual
generators. This introduces additional difficulties compared to the
linear problem, e.g. how to handle noise and uncertainties. Common
tools are quite naturally parameter identification techniques,
elimination theory and state observation techniques.
Publications
Fault isolation
If a fault is detected then the process of identifying the fault is
called fault isolation. Isolation can be achieved by using a
set of tests that are sensitive for different sets of faults. The goal
of our research within fault isolation is to construct a diagnosis
system with the highest possible isolation capability. To obtain this
goal the following issues are currently the topic of our
research: Which set of tests needs to be designed? How are the
decisions of each test defined? and how is the diagnosis statement
computed? To work with these questions formally, a framework for
diagnosis is developed.
Publications
Structural methods
Diagnosis performance regarding fault detectability and
isolability might be required in order to meet for example safety and
environmental protection requirements for the overall process. To be
able to perform model based supervision, some redundancy is needed and
this redundancy can be provided by some sensors together with a model
description of the behavior of the process. Natural questions are what
isolability that can be achieved given a model describing a process
equipped with a fixed set of sensors or if the sensors selection is
part of the problem, to decide which sensors to include in the process
in order to achieve a required fault isolability. For large complex
models, it is suitable to answer these questions using algorithms
analysing only the structural properties of the model, i.e. which
variables that are included in each equation. The development of such
algorithms is a main research topic here.
Publications
Applications
Although our research have a clear theoretical focus, it is of central
importance to keep a high level of industrial relevance in our
research. Thus, application studies are fundamental to get hands-on
experience on how our methods perform in practice and get feedback
when identifying research topics that are both industrially and
academically relevant. Our application studies has predominately been
in the automotive area, mainly because of longstanding collaborations
with automotive industry and our automotive research
laboratory which makes real life testing of diagnostic algorithms
possible.
Publications
Informationsansvarig: Erik Frisk
Senast uppdaterad: 2010-11-29